Five videos portraying a gruesome series of events appeared online in early March. First published by Tigrai Media House, a media outlet affiliated with the Tigray People’s Liberation Front (TPLF), they depict the detention and eventual execution of a group of unarmed men purportedly in the Tigray region of Ethiopia, where a non-international armed conflict has been taking place since November 2020. Under international humanitarian law, the executions depicted in the videos would constitute the war crime of murder. The events were documented by a member of the Ethiopian military turned whistle-blower using a camera phone. Four of the clips show the same location, while the fifth shows a different location with men held captive by Ethiopian troops.
Amnesty International collaborated with the broadcaster CNN to verify and tell the story of these videos, and shed light on these crimes. Despite our best efforts to document the events shown in the videos, we suspected that in the highly polarized context of the Tigray conflict anything we published would likely be dismissed as ‘fake news’. Indeed, a subsequent statement from the Ethiopian National Defence Force (ENDF) dismissed the videos as a fabrication.
Before saying anything publicly, we needed to ascertain who carried out these executions, and where they occurred. We also could not publish without testimony from the families of the victims or eyewitnesses. Working with CNN, we were able to address all of these challenges – as explained in the story published on 1 April.
In this post we explain how we established precisely where these videos were filmed.
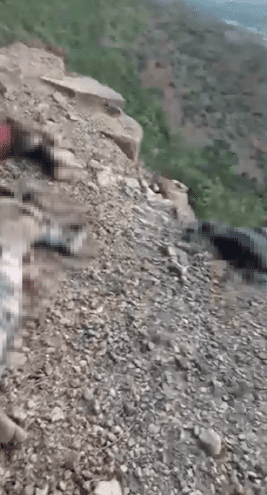
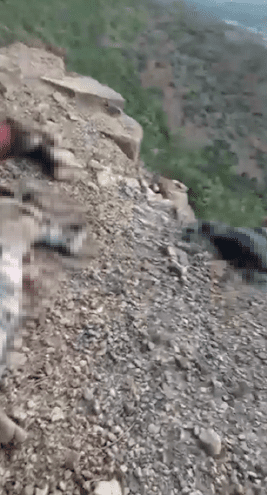
Identifying an area
With any geolocation challenge, the first step is to identify a general region. When the videos first emerged in early March, there were many rumours flying around in the open source community as to where it could be. Eventually, we were able to narrow this down to an area south-east of the small town of Mahibere Dego, 15 kilometres southeast of Axum in the Tigray region. This area is circled below in yellow.
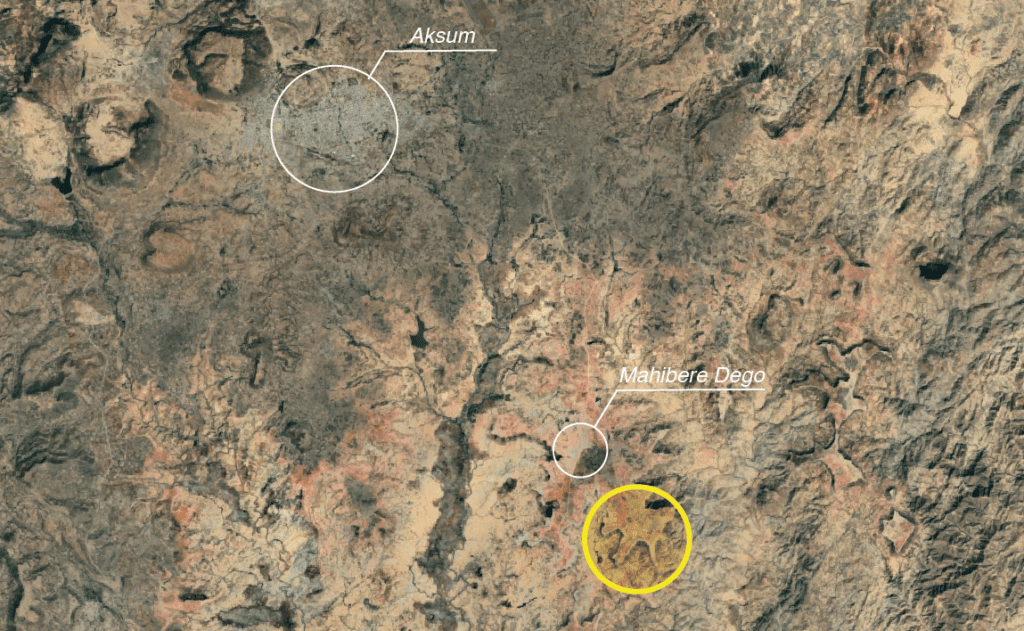
This fitted, as we could see from the videos that the soldiers and their prisoners were pictured on the edge of an escarpment. This area was full of such geographic features.
Using shadow analysis, we were then able to exclude many areas on the plateau upon which Mahibere Dego sits. Analyzing shadows cast by figures in the videos, we were able to see two elements which helped us. Firstly, that the shadows were much longer than the people were tall. We used the tool suncalc.org to confirm that shadows longer than the height of the people meant that the videos were filmed either late in the afternoon or early in the morning. Looking at the sequence of videos, where the later shootings happen with no shadow at dusk, we were able to ascertain that the shootings happened in the late afternoon/early evening. With that information, we were able to conclude, secondly, that the direction the shadows were running was roughly SW-NE.
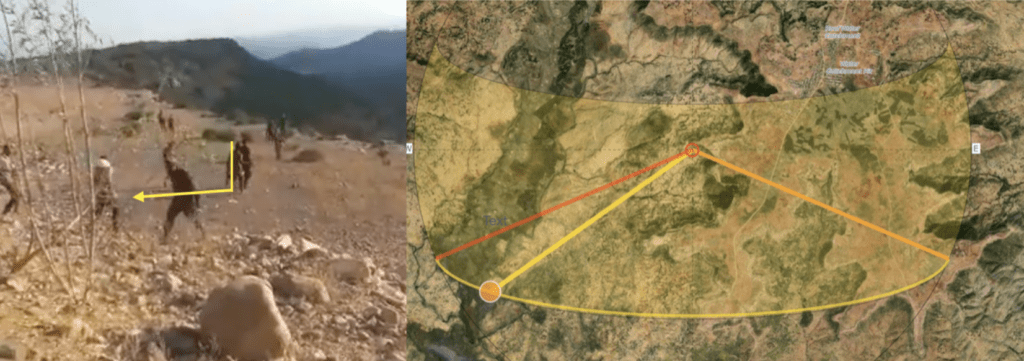
We then spent time studying every escarpment fitting those general features in the area around Mahbere Dago. We identified the area around this location (13.999696 38.788995) as a likely spot.
While our geolocation work convinced us that this general location was correct, we still had doubts because the rendering of the area by Google Earth Pro contained many uncertainties. In order, therefore, to confirm this with precision, we opted to painstakingly reconstruct the area using a 3D model.
In this piece, we outline why we took this approach, and explain the limitations of our 3D analysis and reconstruction.
Uncertainty – inside Google Earth Pro
In visual verification, uncertainty is unavoidable, especially in the cases where it is impossible to physically visit and survey the location. It is particularly important to understand the sources of the uncertainty in the ongoing process of controlling confirmation bias and improving the accuracy and precision of the method used for visual verification.
For the first location, through interrogating the content and comparing it to the ‘terrain’ view option in Google Earth Pro, we identified, in the set of videos in which the executions occur, the characteristic shape – a sort of carving in the surface, resembling a gully or crevice.

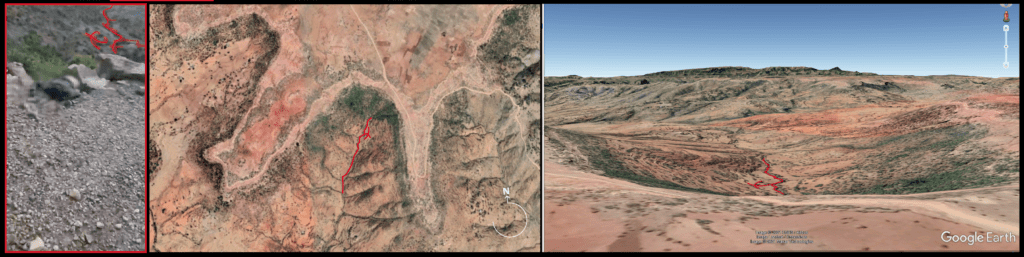
Although the features rendered in Google Earth Pro seem to reflect those visible in the footage, upon referencing the profile of the mountains in the background, certain differences created a lingering uncertainty that gave us cause for doubt.
Confirmation anxiety
This lingering doubt was caused by the inherent flat rendering of the Google Earth Pro mesh. While attempting to match the location of the footage, the rendering quality made it difficult to perceive depth of field and therefore made differentiating mountain ranges from each other more complicated. The greater uncertainty was heightened by the apparent lack of spatial representation of smaller features visible in the texture of the Google Earth Pro landscape – such as mountain peaks, cliff-edges or gullies.
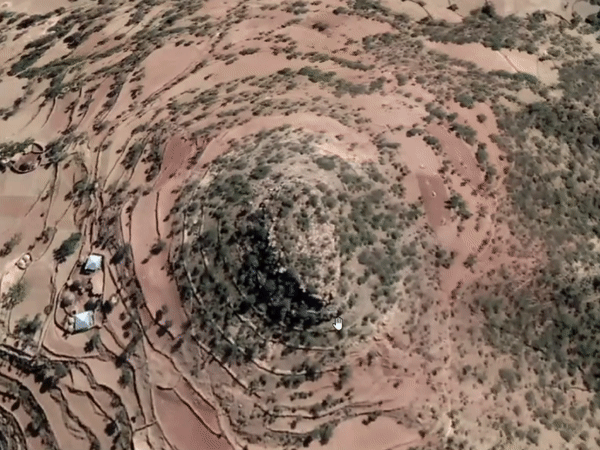
This example above – a screen recording of one of the identifying features for the second location in Google Earth Pro – exposes the difference in the high-definition landscape texture, and the significantly less defined, ‘flattened’ height profile within the underlying height model.
The uncertainty therefore lies between the promise of positive identification occurring on the surface of the Google Earth Pro mesh and the uncanny lack of relative spatial representation of these features in the mesh topology.
This feeling of what we call ‘confirmation anxiety’ is also reinforced by the viewing position and angle of the Google Earth Pro preview. The viewing position in Google Earth Pro is, by default, within a range of approximately between 2 and 10 metres above the terrain, which is considerably higher than the angle from which a human would film using a mobile device. Depending on the scene, therefore, the most minute of changes of a viewing angle combined with a change of vertical camera position can distort or obscure the view captured in Google Earth Pro. The inability to control those parameters within Google Earth Pro’s interface leads to further biases and uncertainty in the process of visual geolocation.
What is Google Earth Pro doing?
Google Earth Pro’s Digital Elevation Model (or DEM) is an aggregate of height information derived from multiple sources. Despite a lack of transparency in communicating the process of how the final DEM is derived for different types of locations, it is known that, especially in ‘non-data’ areas such as in mountains and desert, Google has primarily relied on the topographic data generated from NASA’s Shuttle Radar Topography Mission (SRTM) and then used a custom algorithm to further inform its elevation data. It’s worth noting that, despite Google’s likely incorporation of other digital surface models and the use of LIDAR measurements to provide better elevation accuracy and precision for selected locations around the globe, it is probable that in the case of this location in Tigray, Ethiopia, little work has been conducted to improve height data information. Height data information created the major source of doubt here.
Notably in the various existing comparative LIDAR to SRTM studies, the resulting error has been identified as being between 5 and 10 metres. The study produced by the US National Geospatial-Intelligence Agency argues that the errors vary across continents – and the biggest errors are across Africa, with an absolute error of 5.6 metres.
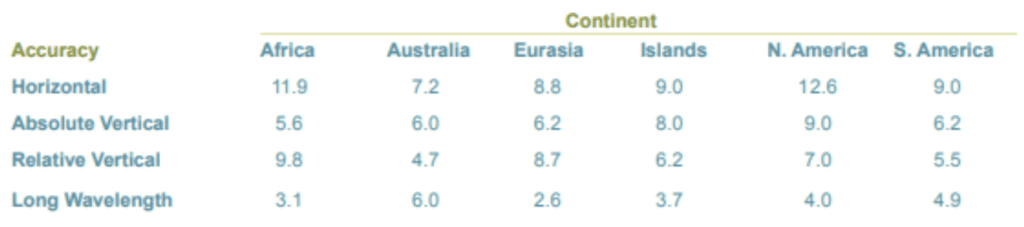
Interrogating the Google Earth DEM
With the plan of spatial reconstruction of the footage within the model, based on Google Earth Pro, we set out to extract the DEM data and integrate it into our 3D modelling software – Blender. We used a technique and an add-on for the Blender application developed by Elie Michel. This add-on integrates captures from Google Earth Web (an online counterpart to Google Earth Pro), using RenderDoc (a frame-capture based graphics debugger), into the Blender environment.
While it is not certain whether the resulting mesh subdivision in Blender reflects the resolution of the information in Google Earth and to what extent it is affected by the process of data extraction, the mesh resolution – characterized by low subdivision – seems reasonably consistent with the level of detail seen in Google Earth Pro’s surface model, as shown in this example:

The above sequence compares the Google Earth Pro preview to the mesh extracted into the 3D modelling software. The subdivision of the mesh is signified by the edges.
The resolution of the mesh seems to be consistent with the precision of the surface model in Google Earth Pro. It also signals that, for this location, any elevation detail below approximately a 65-85 metre resolution is not being picked up.
Spatial integration of Google Earth data and footage – first location
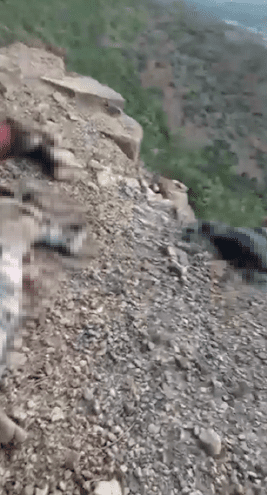
The extracted model was used to establish the first location. The relatively low resolution of the texture of the imported Google Earth Web model was mitigated by projecting a recent high-resolution commercial satellite image of the area of interest back onto the model.
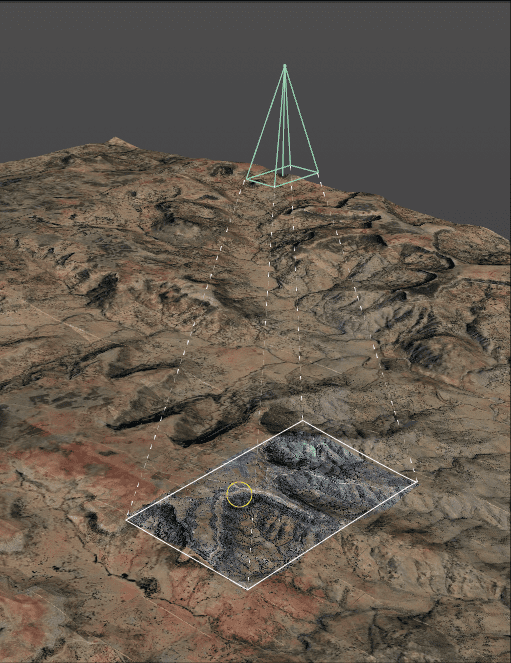
To spatially reconstruct the camera that recorded the key footage of the first location, we used a process of footage tracking in Blender. As a result the camera movement was replicated within the digital space and a focal length for the camera estimated.
Following the spatial translation of the footage, we started the reconstruction by matching the frame in the video sequence that displayed the characteristic ‘carving’ in the landscape most clearly. This match informed the position of the camera in space.
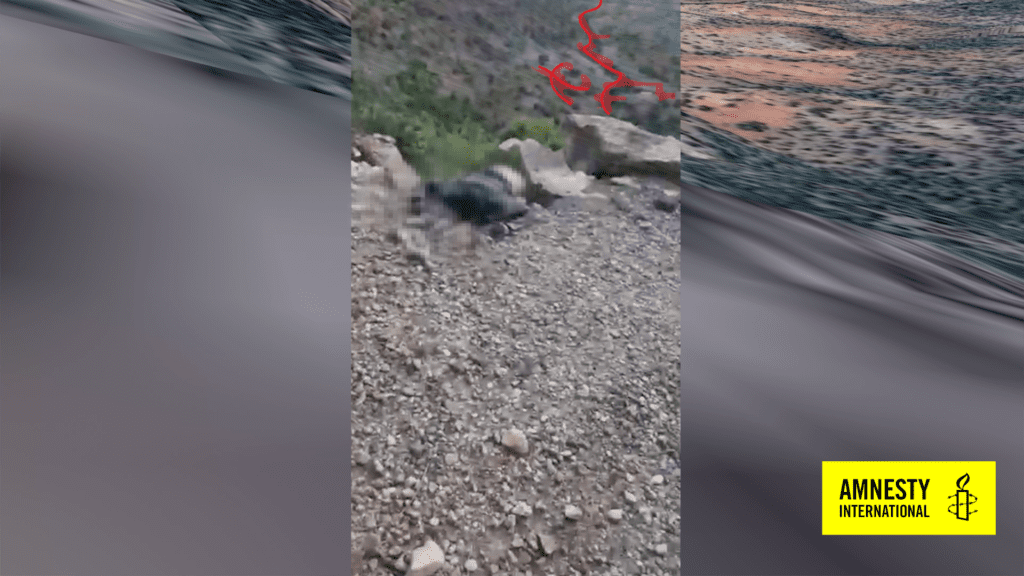
Based on that we could proceed to confidently match other frames in the model.
In this process other characteristic features seen in the image below, such as a curved path in the mountainside (red), a denser patch of greenery on the top of the hill (green) and the profile of the mountain in the background (blue), were marked.
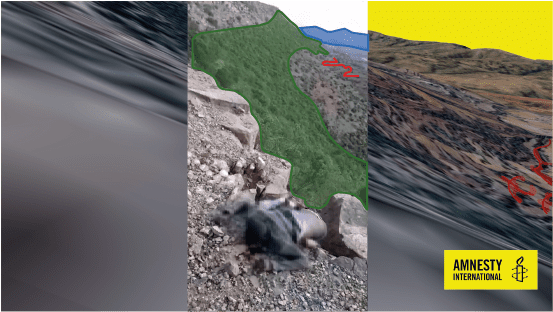
By projecting these simplified graphics from the established camera positions onto the landscape, the information from the footage begins to form reference points.
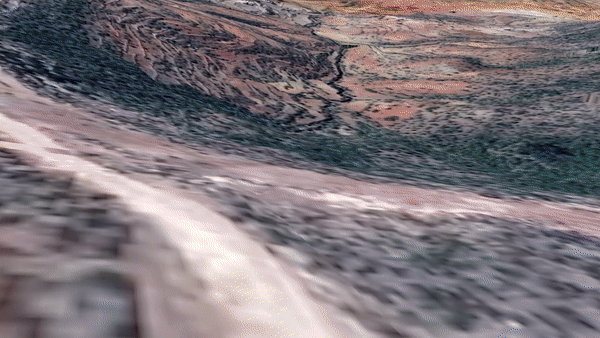
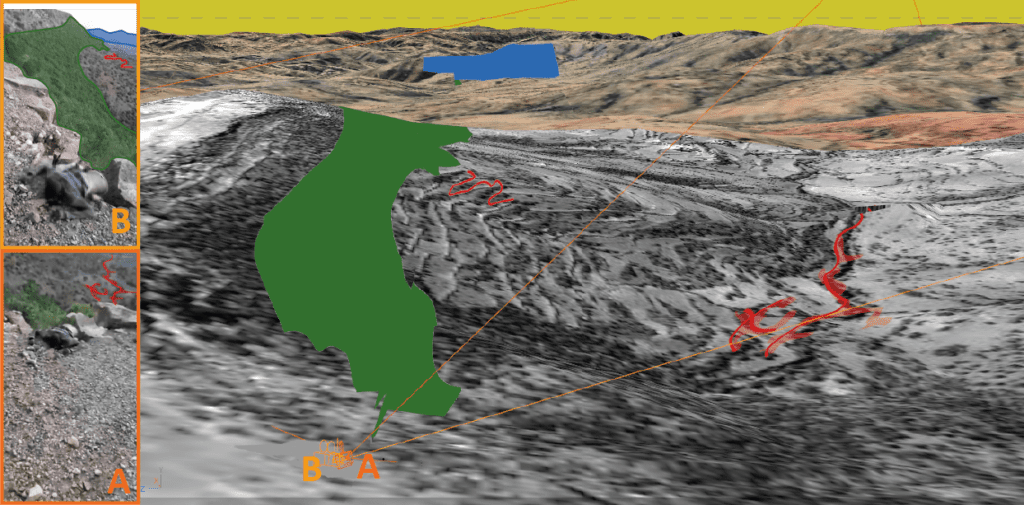
Upon mapping the footage within the model, it became clear that the features in the background and foreground matched the landscape, yet there was still cause for doubt as they did not match completely.
The the red outline projected on top of the landscape, for example, did not precisely overlap with the outline of the carving (as illustrated in the example above). This could result from a number of factors – including lapses in the Google Earth Pro mesh or the low resolution of the elevation data erasing the nuance of the topology around the depression in the landscape. In general, given these known limitations, seeking higher precision for the camera location is not necessary to establish the general location. For our geolocation purposes we needed to ensure this wasn’t caused by an error in the camera matching, as we set out to map the rest of the footage onto the landscape as accurately as possible and identify other features in the process.
As a next step, we set out to include more reference points in the landscape and model the detail of the foreground based upon these points. We identified small features of landscape captured in the satellite imagery. We suspected that the characteristic darker patches would most likely be representations of bushes or other small-scale objects or features.
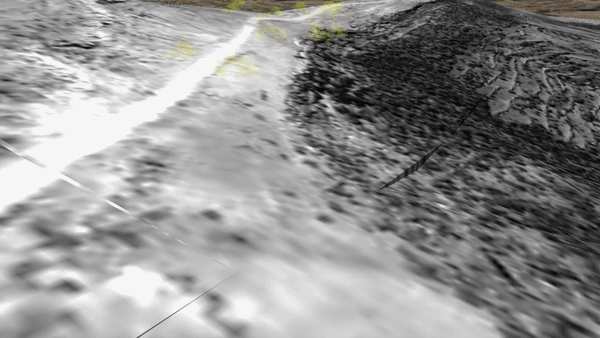
Models representing bushes, similar to the ones seen in the footage, were placed in these spots. The continuing fragments of tracked footage matched with the position of the bushes. For further verification, we also placed models representing the victims of the massacre themselves into the model.
Based on our improved match, we could assess the difference in the mesh extracted from Google Earth Pro and the edges and rocks seen in the foreground at the start of the footage:
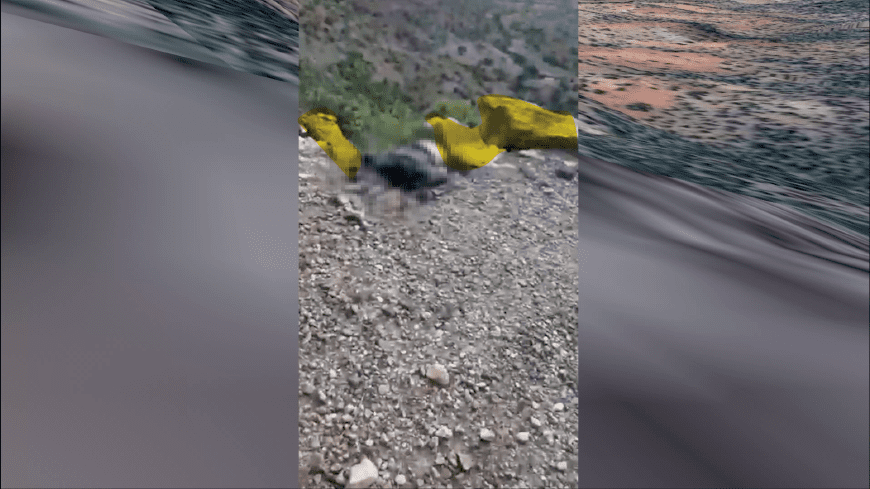
Doing this allowed us to estimate the difference in the elevation of the ground around the location, both in reality and in Google Earth Pro. The difference was approximately 2 metres – less than the known absolute error of 5.6 metres assumed for the purposes of this reconstruction.
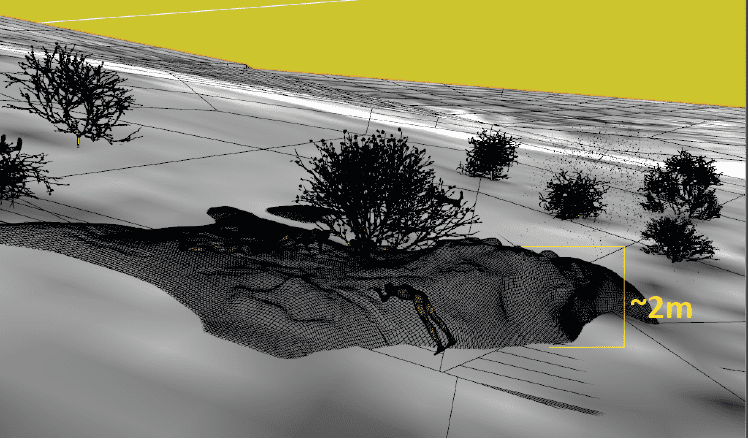
Importantly, the reconstruction of the foreground allowed a more confident mapping of the second part of the footage in the model space. This further matched the features seen in the imagery background – the cliffside, which was another important component of the geolocation process.
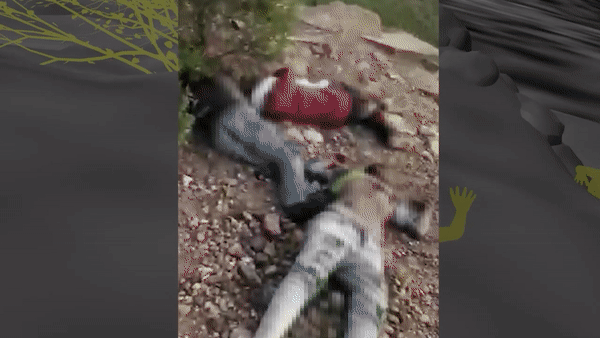
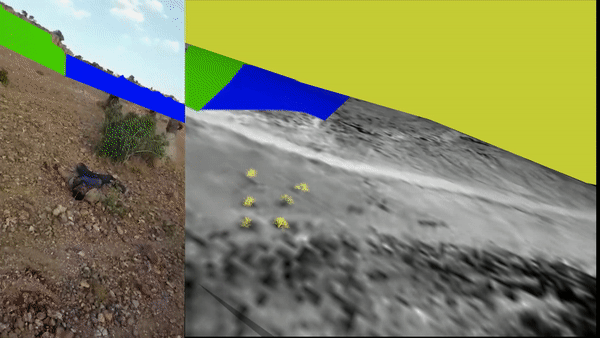
Spatial analysis use in matching the second location
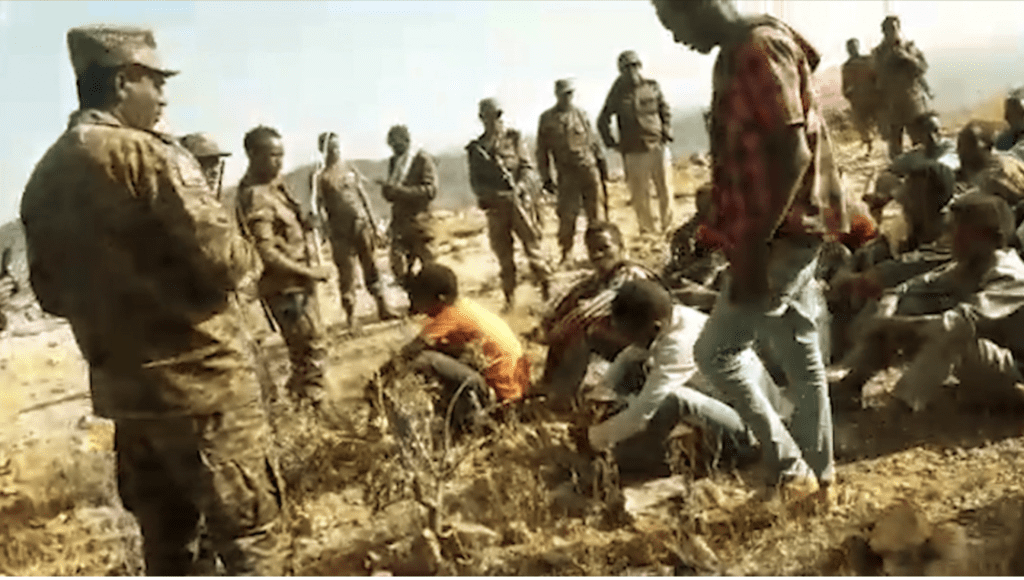
In the case of the footage from the second location, the primary clues that indicate the general area concerned the outline of the mountains seen in the background:
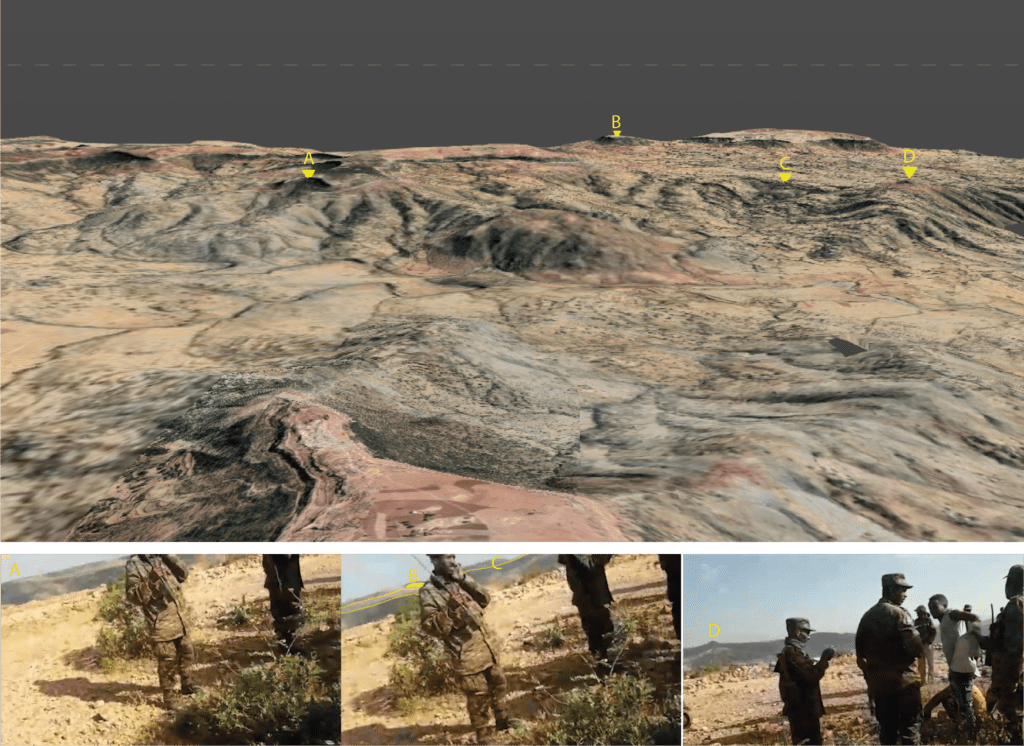
Despite lack of other identifiable or unique features in the foreground, what we can see is that the location at which the video was captured is close to the edge of a hill or cliff. By repeating the process of footage tracking and camera reconstruction, we began placing people in the scene. This helped us discern that the distance between the videographer and the edge of the cliff is between 20 and 25 metres at least.
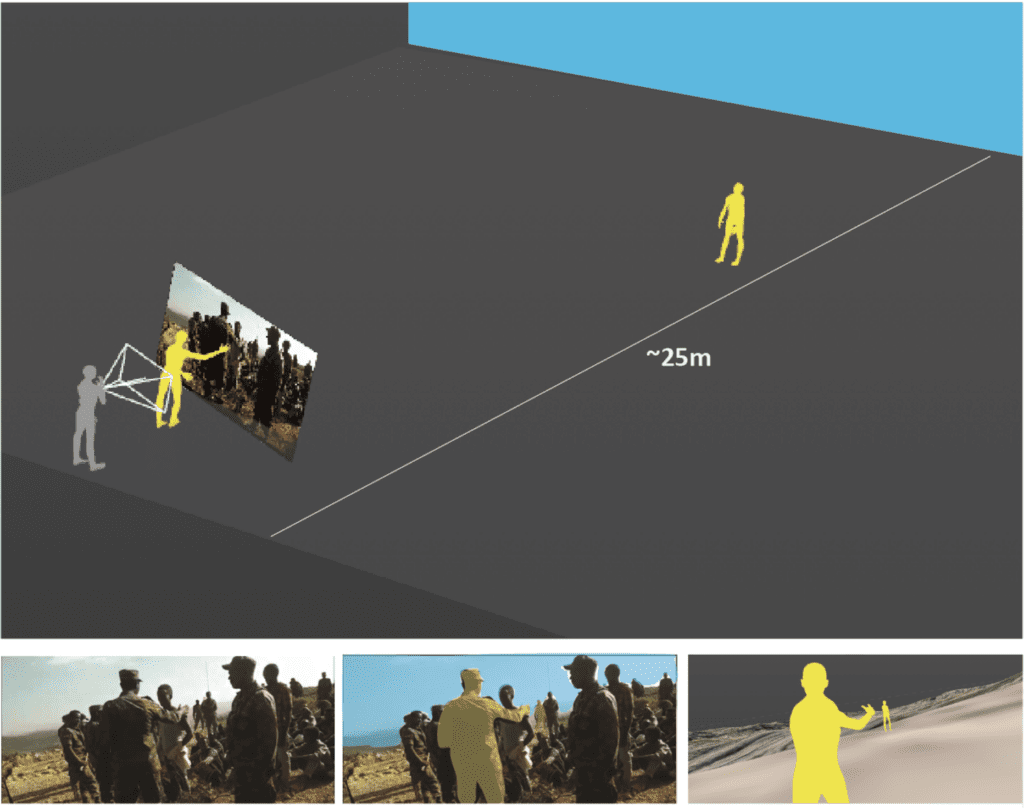
Looking at various possible locations that corresponded to this dimension in the model, we could see in the location marked here that a path comes to an end:
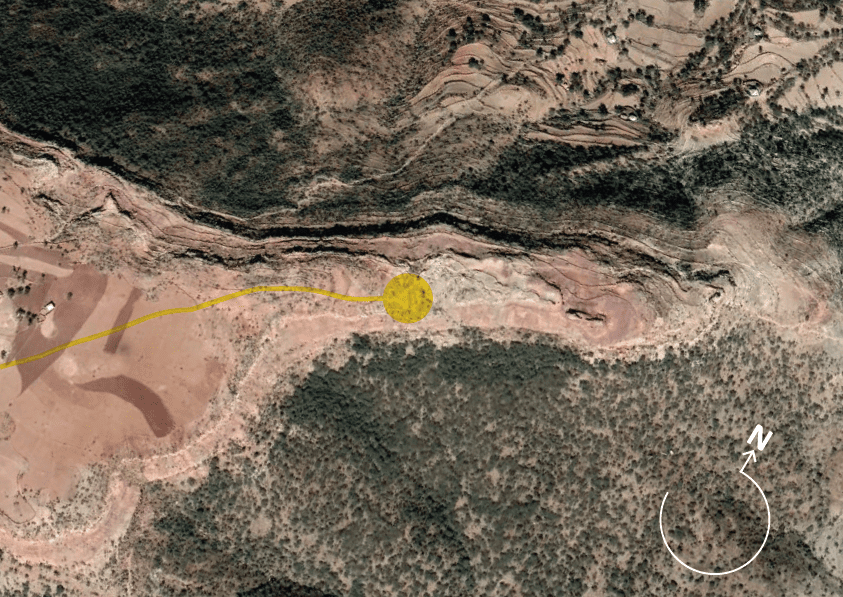
Therefore we suspected that the footage could have been taken from this general area. Similarly to the processes described previously – we placed a tracked camera for a segment of the footage in this location. The background in the model matched the footage, yet given the distance to the matching objects we were conscious of possible error.
Knowing the limitations of the resolutions of both the video and the mesh, we tested limits of the possible locations that, within the permitted margin of elevation error in the base model (of 5.6 metres), matched the features seen in the footage. The resulting location estimation has a higher margin of error than the first location’s geolocation – a lower confidence that needs to be communicated clearly in the investigation.
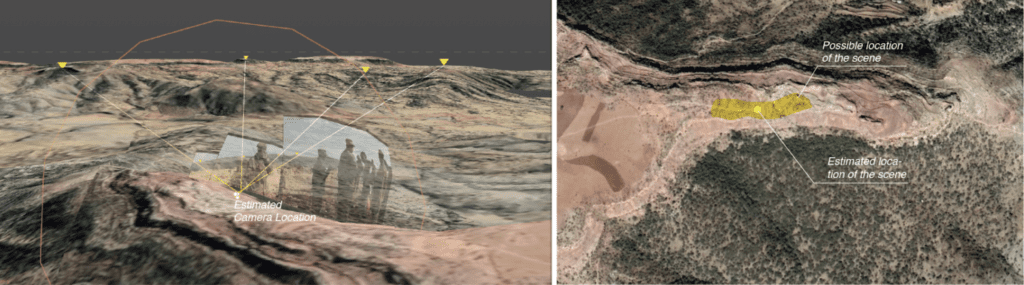
Comparison between visual and spatial reconstruction approaches
BBC Africa Eye, Bellingcat and Newsy published reports on the same footage. Through their reports we see that the same problems were encountered independently by both investigation teams. When comparing our estimated locations to the location estimated by the other team, there is a difference of circa 250 metres.
The BBC Africa Eye, Bellingcat, Newsy team attempted to clarify the uncertainty produced by the qualities of Google Earth Pro by seeking another form of representation of the hillsides by using Peakvisor.
Peakvisor renders the mountains in a different way than Google Earth Pro, using a dataset for elevation that includes ALOS World 3D and Viewer Finder Panoramas. The resolution of height seems slightly improved in certain locations and the graphic representation clearly distinguishes outlines of the mountains with a continuous black line and labels for peaks. However, spatially the lapses seen in Google Earth Pro are repeated. For this particular location, the process behind generating elevation data remains opaque and the camera’s relationship to the terrain is again difficult to establish.
When interrogating the location estimated by BBC Africa Eye, Bellingcat and Newsy, at a first glance we see that the slope in the suggested location is much steeper and hence not likely to fit the scene. However, since the satellite image in the location suggests a cliff – consistent with the rest of the area, it is possible that Google Earth Pro’s elevation error is considerably higher here than at other spots around the site. This poses a challenge for our spatial verification method. In order for this location to correlate to the spatial conditions seen in the footage, we would need to manipulate the mesh outside of the assumed margin of error, with little indication to its real conditions given the limited scope of the visual evidence.
This highlights the need to keep questioning the possibility of greater lapses of accuracy within Google Earth Pro and understanding their possible roots relative to other topologies and locations.
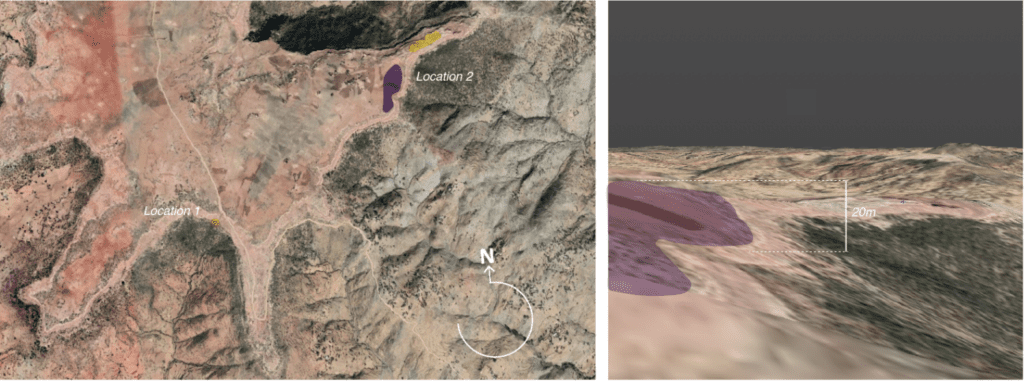
The value of spatial reconstruction for geolocation
It is important to underline that such high precision was not necessary here – an understanding of the general location of where this video sequence happened was what was required to speak to it – as both investigations did. Indeed, it would arguably have proved to be counterproductive to spend countless hours trying to calm any lingering confirmation anxiety.
However, this unique opportunity for a comparison of two approaches to geolocation not only highlights the need to always confront the accuracy and precision of Google Earth Pro’s DEM, but also provides interesting strategies for secondary verification.
The goal in this post was to speak to both the limitations and advantages of reconstructing and inhabiting a scene through 3D software, while interrogating the limitations of conventional geolocation methods and tools.
Despite the limitations of the reconstruction method, in this case it was key to mitigate the uncertainties and doubt inherent to the visual geolocation process. Indeed, this work is part of an ongoing process of building improved methodologies and ever-more accurate representations of remotely investigated scenes, as well as producing a catalogue of techniques to apply to the particularities of specific sites and visual materials.